The thought of using data and models to guide turfgrass management decisions may seem daunting, but golf course superintendents have been using both to improve course conditioning for centuries. Superintendents are incredibly observant, and data are simply a set of recorded observations. These data can help us understand what happened in the past and allow us to make predictions about future outcomes.
An early example data-driven turf management occurred after Old Tom Morris spilled sand on the 10th green of Prestwick Golf Club. He spread the sand pile across the green and noted the improved turf vigor six weeks later. After he repeated this process across the course, Old Tom created a mental model that linked sand topdressing to improved turf health and putting green quality (Read More). It was Old Tom’s careful observations and his mental model that led to the development of regular sand topdressing. Today, it’s easy to collect a lot of data, but data alone needs to be interpreted with a model to aid decision-making.
Demystify Modeling
Not all models are complex. Here’s another example; a golf course superintendent sees wilting turf. The superintendent predicts that hand-watering will correct the wilt based on their past observations and experience. That simple mental model may be correct and the wilt recovers, but sometimes models are wrong. Models provide a best guess or probability that something will or will not occur. Maybe there is a root disease or or the soil is hydrophobic and the hand-watering does not correct the wilt. Additional data points including measurements from a moisture meter or past wetting agent records may help understand why the wilt did not improve. This additional data required a more complex mental model to offer alternative solutions to help solve the problem.
There have been a lot of new technologies in the turf management arena to collect data, but it may not all be actionable – at least at the moment. Data including the speed of the golf carts or the average golfer handicap won’t help solve the wilting turf problem. Data is only valuable when it improves the accuracy of a model to drive a management decision. The hand-held moisture (TDR) meter is an example of how data could immediately improve management efficiency. Now managers could quickly measure soil moisture, run it through a mental model (how near is the reading to the expected wilt point) and decide if water is required immediately, later this afternoon, or tonight.
Automation Expands complexity & ease
Drones, mower-mounted sensors, weather station networks, GPS sprayers, and even handheld devices like cameras, moisture meters, stimp meters and clipping volume collection buckets can generate a slew of new data (written observations). Automatically turning these data points into management recommendations can dramatically improve decision-making. Without automation many of these new data points can be more work than help.
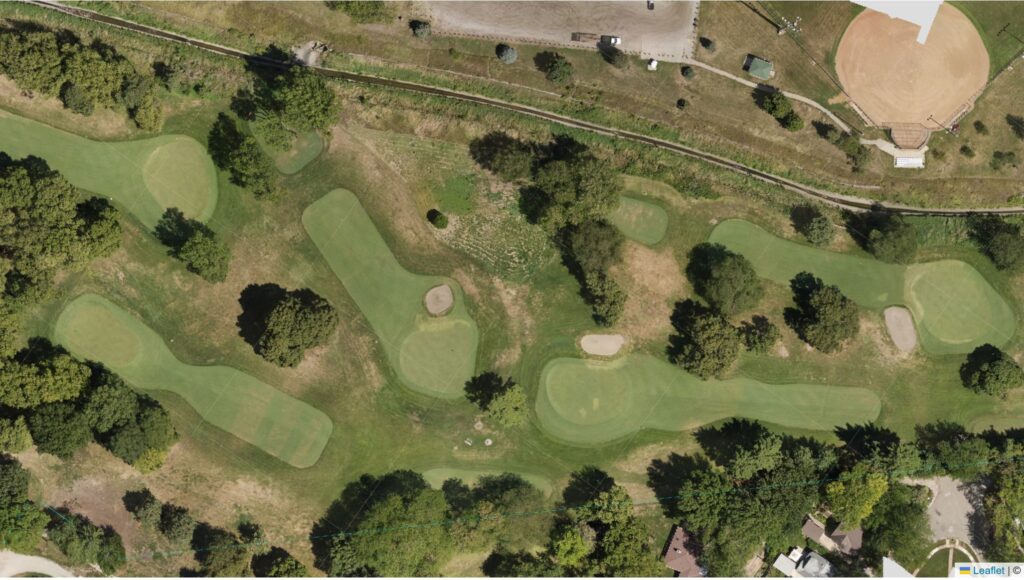
Decision support tools like GreenKeeper App are data-repositories designed to simplify the task of logging and analyzing data being generated at a golf course. Some guidance is simple including mixing math, weather and application record-keeping, and storage of turfgrass performance data including green speed, growth rate (clipping volume), soil moisture, etc. GreenKeeper App then uses proprietary models to make predictions about future events. Growing degree day models can predict PGR and DMI growth suppression, soil organic matter models can help predict organic matter accumulation in golf turf, and pest models can help superintendents stay ahead of pest problems.
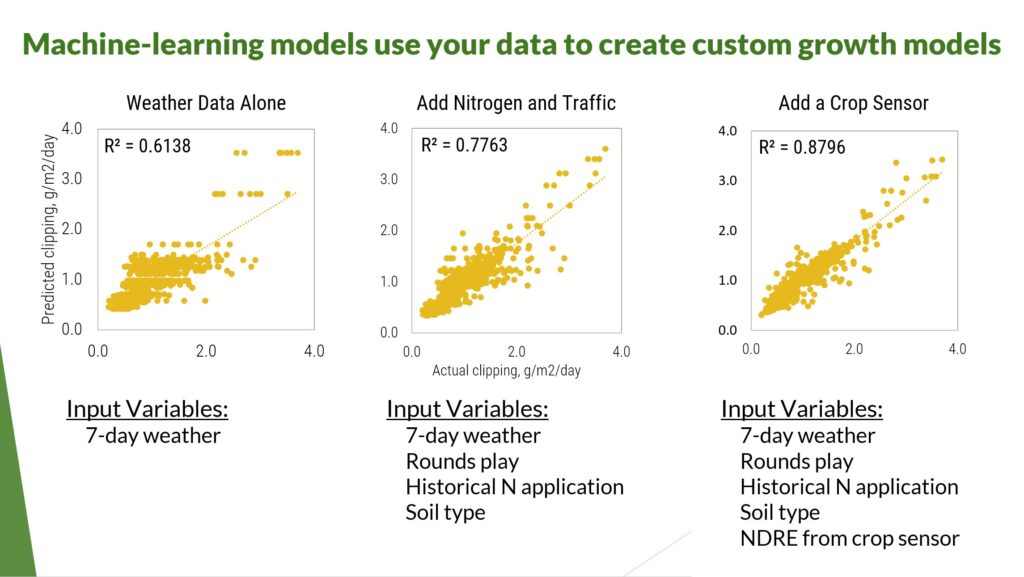
In 2023, GreenKeeper App is using machine learning algorithms to generate local models from a course’s own data to predict growth rate, green speed, and pest outbreaks. Advanced computer programs find connections between different factors like management practices, weather and application records, and sensor data to generate different types of models that are specific to individual golf courses. These models will then provide predictions that can help golf course superintendents stay ahead of their course conditioning.
In the subsequent series of blog posts, I’ll dive into ways to collect, organize and interpret data with GreenKeeper App. Topics will include growth rate manipulation, different ways to schedule nitrogen fertilizer, low-tech but actionable metrics to use today, predicting soil organic matter changes after cultivation, and high-tech solutions to improve sustainability. Use GreenKeeper App to start collecting data at your course this season and gain access to advanced models based on your own data. Sign up for a free trial (no credit card required) at GreenKeeperApp.com.